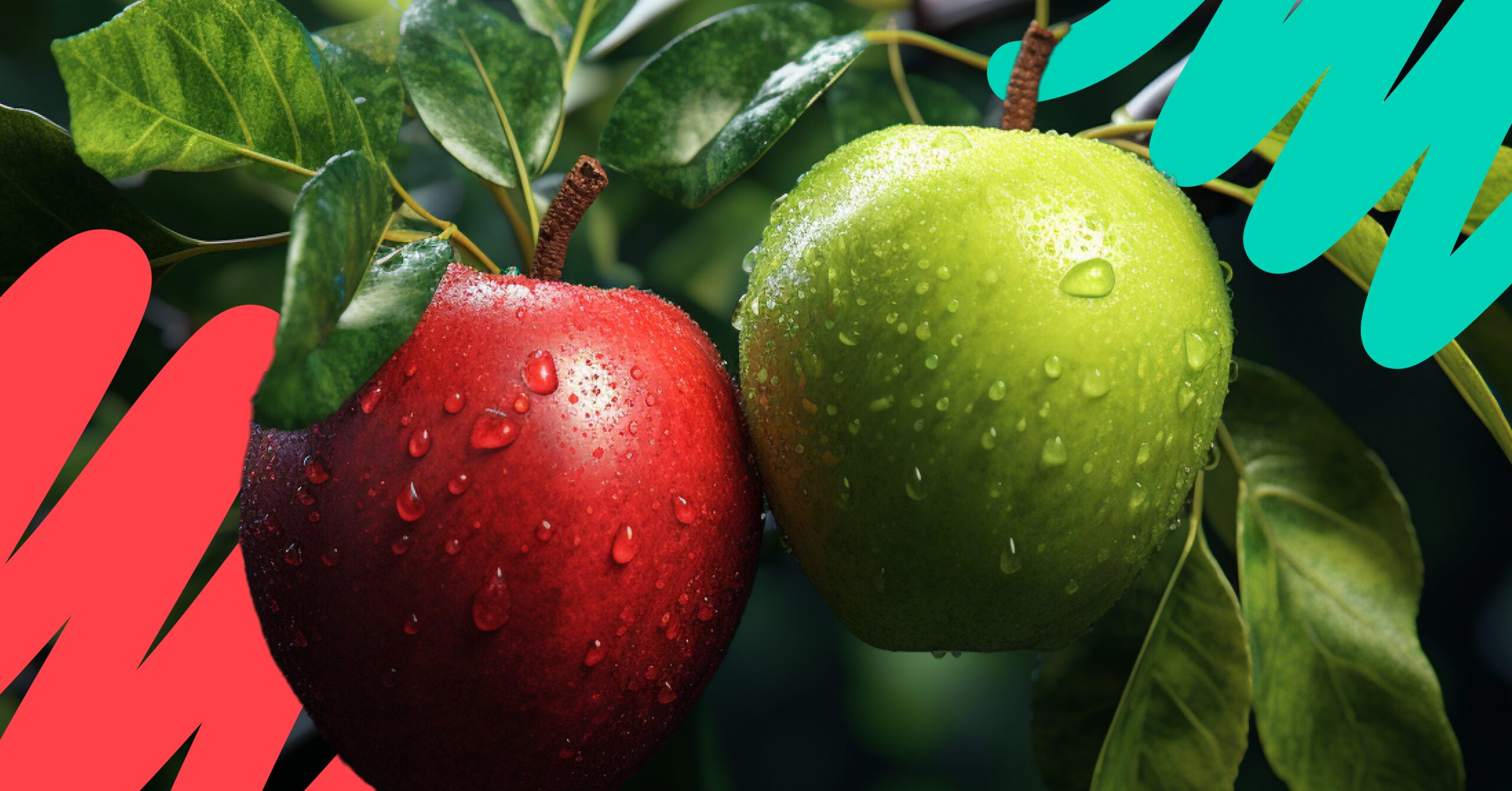
Despite all the wonderful things artificial intelligence (AI) appears to be able to do for us, we should remember that these systems are only tools. They are not ‘intelligent’ as we humans measure intelligence, and Large Language Models (LLMs) in particular—my focus for this article—are, at root, regurgitating variations of the input they have been trained on.
One of the key things missing from any of these AI tools is anything like a sense of ethics. They have been trained on the vast ocean of words found on the public internet, but they don’t have a human ability to distinguish between content that reads well, and content that is ethically dubious.
Understanding AI ethics
The concept of ethics in the field of artificial intelligence (AI) has gained significant importance in today’s rapidly evolving technological landscape. The incorporation of ethics in the use of AI is crucial to ensure that the content created with these tools reflects the ethics of an organisation, and of society more generally.
Any discussion of AI and ethics must be multifaceted, as ethical issues include everything from data responsibility and privacy, fairness, and transparency, to environmental sustainability, inclusion, accountability, trust, and technology misuse.
It is often the case that ethical violations are made unintentionally. When the primary goal of a tool is improving commercial outcomes, oversight and unintended consequences can follow, “particularly due to poor upfront research design and biased datasets”.
As with any emerging technology, unforeseen harms can come to light along the way. Regulatory frameworks need time to catch up, so the onus of ensuring ethical considerations are made must, for now, be on the creators of novel AI systems.
The need for transparency
There is an increasing concern that the creators of the leading AI technologies must provide a level of transparency about their tools. They are being encouraged to open up about the data the LLM are trained on, for instance.
Transparency should be present at all stages of a project’s development, with responsibility not limited only to the developers building the code that powers the system. It is important to make AI systems’ functions and decision-making processes clear and comprehensible, so we can gain an understanding of the biases built in to the systems we use and how to avoid them.
“Transparency is a chain that travels from the designers to developers to executives who approve deployment to the people it impacts and everyone in between. Transparency is the systematic transference of knowledge from one stakeholder to another: the data collectors being transparent with data scientists about what data was collected and how it was collected and, in turn, data scientists being transparent with executives about why one model was chosen over another and the steps that were taken to mitigate bias, for instance.”
Reid Blackman & Beena Amanaath, Harvard Business Review
As well as being crucial for building trust among users, maintaining a focus on transparency can mitigate reputational, regulatory, legal and commercial risks faced by businesses; avoiding these costly penalties can add weight to the already significant moral arguments for showing your working.
Bias and fairness in AI
Algorithmic bias in AI systems refers to the tendency to produce results that are systemically prejudiced due to flawed assumptions in the machine learning process. As more and more content is produced with the help of AI tools, understanding and implementing AI ethics becomes increasingly vital to maintain trust and accountability.
This understanding will foster trust and confidence in AI technologies, especially in sectors where AI decisions have significant implications or can lead to unfair outcomes, such as job recruitment, credit scoring, healthcare, finance, and law enforcement.
Some systems have already proven to produce dubious results, especially when those results are taken at face value rather than reviewed critically by humans.
Demonstrating the real-world risk such bias can pose, research has found that autonomous driving systems are 20% worse at recognising children than adults, and 7.5% worse at recognising darker-skinned than lighter-skinned pedestrians. The issue is believed to stem from the image data used to train the autonomous driving model.
Another example of the ways in which the tools can exhibit the biases built in to their training material is in the generation of images. Several of the popular image generation tools will reflect the common, often unconscious, biases of their training material. Producing images that reflect a one-sided Westernised view of the world, such as Stable Diffusion’s text-to-image model, which was found by one study to consistently favour white-skinned men over people of colour and women.
For more information and a deeper dive into understanding bias in facial recognition technologies, this explainer from The Alan Turing Institute provides a comprehensive look at the context and development of ingrained biases.
Another recent example of the risks associated with unmitigated AI bias comes from the UK, where AI tools used across the public sector have faced accusations of discrimination. The systems in place are perceived to have disproportionately affected non-UK nationals negatively. The same report notes that facial recognition technology in use by a major UK police force “falsely detected at least five times more black people than white people”.
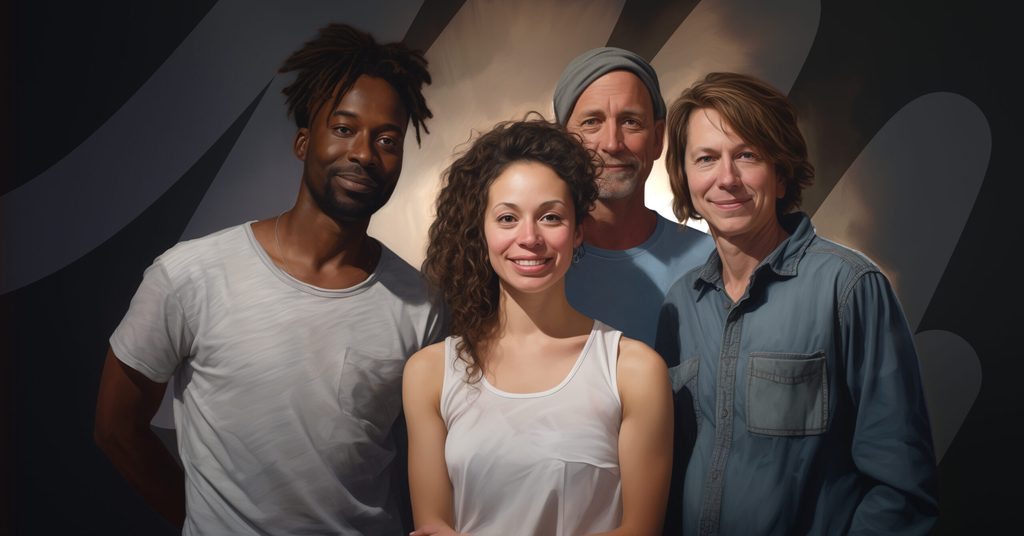
Mitigating and preventing bias in AI
To prevent algorithmic bias, it is essential to use diverse and representative datasets during the AI training process, and to regularly audit AI systems for bias. Again, this is something that requires the aforementioned transparency, and, as discussed, the stakes could not be higher.
In 2021, UNESCO published the first-ever global standard on AI ethics, recognising the importance of human oversight of AI systems. The framework places the protection of human rights and dignity at the centre, with particular attention paid to avoiding the ingraining of existing biases.
“In no other field is the ethical compass more relevant than in artificial intelligence. These general-purpose technologies are re-shaping the way we work, interact, and live. The world is set to change at a pace not seen since the deployment of the printing press six centuries ago. AI technology brings major benefits in many areas, but without the ethical guardrails, it risks reproducing real world biases and discrimination, fueling divisions and threatening fundamental human rights and freedoms.”
Gabriela Ramos, Assistant Director-General for Social and Human Sciences of UNESCO
Even where transparency within some larger organisations is slow to appear, one would hope the development of AI would involve diverse and multidisciplinary teams to ensure various perspectives are considered.
Privacy and data protection
As AI technologies often rely on large amounts of data for their operation, it’s crucial to ensure that any personal data is handled responsibly and securely. Misuse or mishandling of such data can lead to privacy breaches and loss of trust.
If you are building systems trained on your customer data, for instance, you must implement robust data protection measures, including encryption and anonymisation. Without these measures, the risk of personal data leaking into the output of these systems increases.
Ideally, your AI systems should be designed to respect privacy by default and by design. They should collect and use only the minimum necessary data.
The ethical handling of personal data by AI systems is not just a legal requirement in most parts of the world, but also a fundamental aspect of maintaining public trust in your organisation.
Accountability
If AI tools are allowed to operate autonomously, making decisions or calculating outcomes, for example, it might seem challenging to assign accountability.
However, it is important to remember these tools are just tools, and responsibility for any consequences of their use lies with the organisation. Any harm or mistakes as a result of using AI tools cannot be blamed on the tools themselves.
Clear guidelines and regulations must be established to determine liability in cases of AI-induced harm or error. It is essential to ensure that there are mechanisms in place to hold the right entities accountable, be they users, the AI developers, or system operators. And ultimately, the buck stops at your organisation.
Requiring those mechanisms be in place will not only help foster trust in AI systems, but will also promote the development of safer and more reliable technology.
AI national and international guidelines and regulations
There are some national regulations and guidelines around today, though not always very comprehensive in my opinion. They cover a range of issues, including privacy, transparency, accountability, and fairness, but most have not been designed specifically for AI use cases. The European Union’s General Data Protection Regulation (GDPR), for instance, provides stringent rules for data protection and privacy. Though without any specific clauses around AI, the regulation applies to AI software, and its use too.
Further, guidelines like those developed by the OECD or IEEE emphasise principles such as transparency, fairness, and human oversight in AI systems. However, the rapidly evolving nature of AI technologies means that these guidelines and regulations will always be playing catchup and need to be regularly updated and reinforced with practical mechanisms for enforcement.
Regulators may be on the back foot with technology moving so swiftly, but some local laws are already in place, such as New York City’s Local Law 114, which came into force in April 2023; it requires bias audits of automated tool technologies used by New York City employers, and means employers and employment agencies are liable for the decisions made by AI tools, even if provided by third-party vendors. Furthermore, the EU is aiming to publish the world’s first comprehensive AI law, the AI Act, before the end of 2023.
On that front, the Bletchley Declaration, published after the International AI summit in November 2023, seems to be heading in the right direction. I note it seeks to “strengthen efforts towards the achievement of the United Nations Sustainable Development Goals” and acknowledges “the potential for unforeseen risks stemming from the capability to manipulate content or generate deceptive content.” The Declaration notes that “all actors have a role to play in ensuring the safety of AI: nations, international fora and other initiatives, companies, civil society and academia will need to work together.” As a forum involving over 40 countries which resolves to “sustain an inclusive global dialogue”, it is likely to have significant influence on global legislation over the coming years and will next convene in 2024.
While legislative progress may be underway, in my opinion, it is better to define and implement your own ethical guidelines and mechanisms for now; perhaps influenced by the intentions of the national and international bodies, but without waiting for the necessarily slow processes of governments.
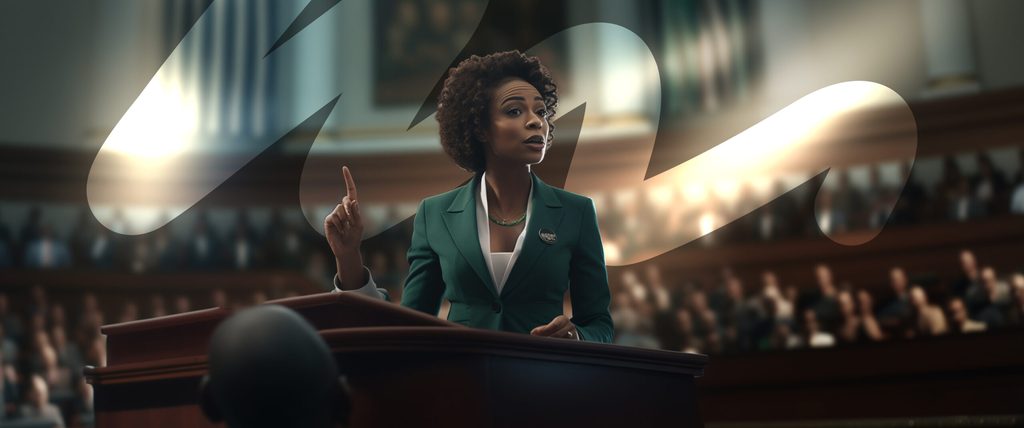
As the world waits for legislation and global protections against the worst harms of AI, it behoves organisations to resist a race to the bottom; taking a humanistic approach to technology benefits both business and society, and will engender the trust needed for widespread adoption.
We’re here to provide advice and support on embedding AI within your organisation while keeping people at the centre. Get in touch with our experts to find out how to create AI workflows that work for everyone.
Ready to reinvent the way you work?
Realise your company’s objectives faster with AI. Reach out to us to discover how our bespoke services can transform your workflows and your business.
Ready to reinvent the way you work?
Realise your company’s objectives faster with AI. Reach out to our team in EMEA to discover how our bespoke services can transform your workflows and your business.
Ready to reinvent the way you work?
Realise your company’s objectives faster with AI. Reach out to our team in APAC to discover how our bespoke services can transform your workflows and your business.
Ready to reinvent the way you work?
Realise your company’s objectives faster with AI. Reach out to our team in the Americas to discover how our bespoke services can transform your workflows and your business.